
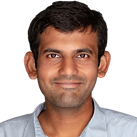
Praneeth Reddy Sudalagunta
Data Scientist. Cancer Researcher. Aerospace Engineer.
Molecular Feature Extraction and Ex Vivo Regression Tree Modeling to Predict Clinical Response to Therapy

Multiple myeloma is characterized by biological heterogeneity between patients, which can lead to heterogeneity in response to therapies. Traditional one-size-fits-all approaches often fail to achieve optimal outcomes, thereby, highlighting the need for more precise treatment strategies. Predictive biomarkers offer a way to match therapies to the individual biology of a patient’s tumor. Molecular profiling of individual patient tumors have the potential to predict the tumor state that is associated with favorable outcomes to a given therapy. To this end, gene expression profiles from RNAseq of patient tumor cells are used to predict functional ex vivo responses to drugs using regression tree models. We leveraged our biomarker discovery tool to identify predictor variables for gene expression, a key step in feature engineering that reduced the number of predictor variables from tens of thousands to a few hundreds. The regression tree models are trained on the median expression of gene clusters identified from the biomarker discovery tool as inputs and ex vivo response AUC as outputs. Such a regression tree model can be used to estimate the ex vivo AUC of any MM patient with RNAseq data. These predicted ex vivo AUCs are used as classifiers to identify MM patients with favorable outcomes.
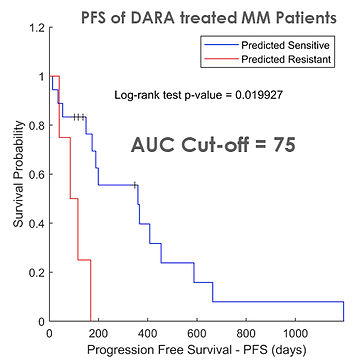
We trained a regression tree model to predict ex vivo AUC to daratumumab in a cohort of 22 MM patients using their RNAseq data obtained shortly before being treated with a daratumumab regimen in the clinic. MM Patients whose predicted AUC is less than or equal to 75 had a significantly longer PFS on a daratumumab containing regimen than those that had higher AUCs predicted by the model. This shows a real-world application of using machine learning techniques to inform patient treatments in the clinic.